OpenAI o1横空出世,让我们看看都有哪些训练大模型的代码开源数据集
OpenAI o1强力袭来
当地时间9月12日,OpenAI正式发布OpenAI o1。全新命名的o1系列包含了OpenAI o1、OpenAI o1-preview和OpenAI o1-mini三款模型版本,相比之前的模型更能胜任科学、编程和数学方面的复杂任务推理和解决更困难的问题。
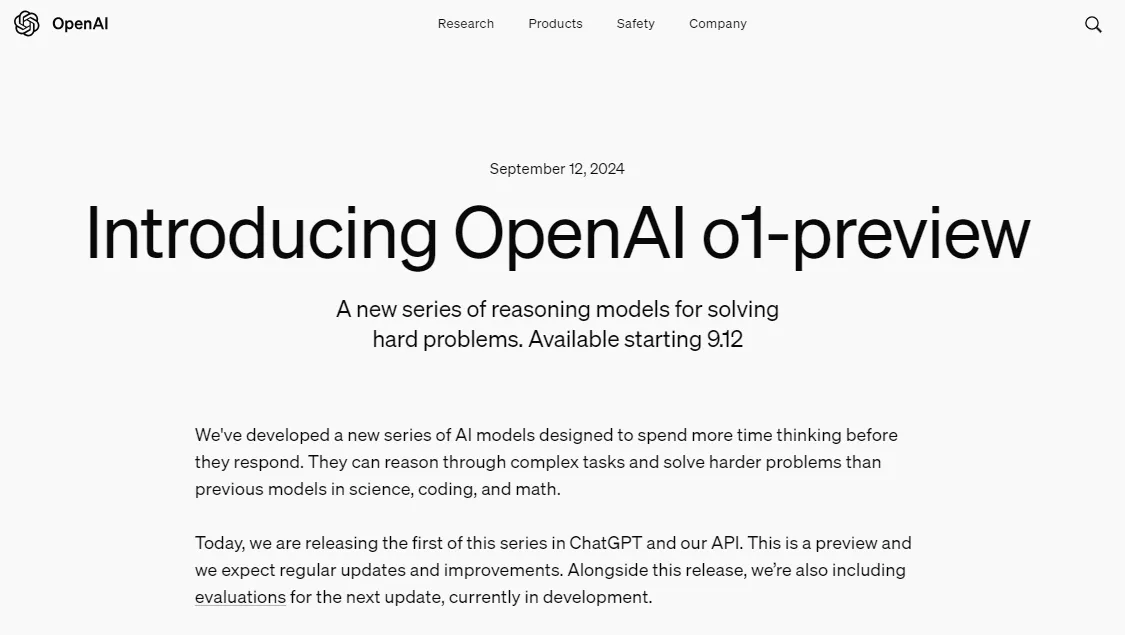
OpenAI官网介绍OpenAI o1-preview
在OpenAI的测试中,新模型在物理、化学和生物学的具有挑战性的基准任务上的表现与博士生水平相似。它在数学和编码方面表现出色,在国际数学奥林匹克 (IMO) 资格考试中o1模型准确率高达83%,在编程比赛测试(Codeforces)中达到了89%的百分位,并在博士难度的科学问题测试中以78%的准确率超过人类专家。
o1 在具有挑战性的推理基准上显著优于 GPT-4o
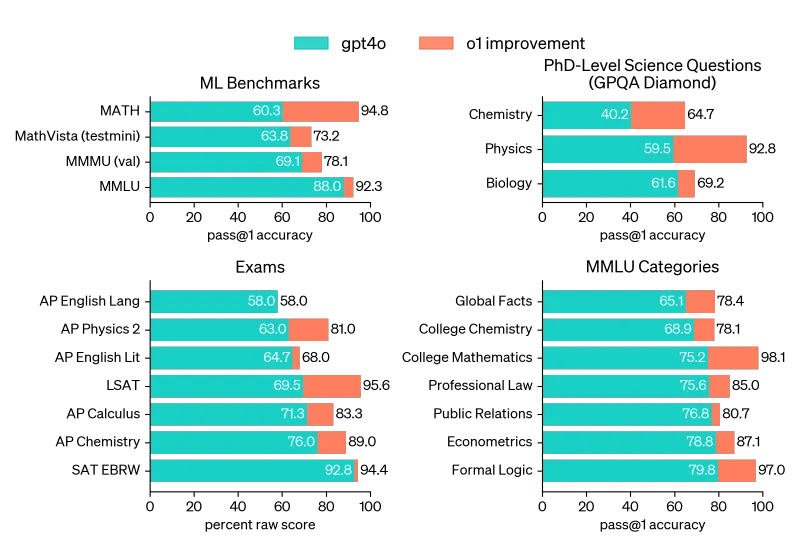
o1 在广泛的基准测试中都比 GPT-4o 有所改进,包括 54/57 个 MMLU 子类别
思维链
OpenAI o1是一种新的大型语言模型(LLM),经过强化学习训练可以执行复杂的推理。此前,大模型常因无法进行结构化推理而受到批评,这主要因为它们依赖于非结构化的文本数据,这些数据缺乏严格的逻辑和规则,导致模型更擅长生成语言,而不是进行逻辑推理或遵循固定规则。为了解决这个问题,OpenAI提出了使用思维链(Chain of Thought, CoT)的方法。OpenAI o1 在回答之前会思考——它可以在回应用户之前产生一个长长的内部思路链。o1模型在密码、编程、数学、填字、英语、科学、安全和健康等领域已经能够通过思维链呈现。
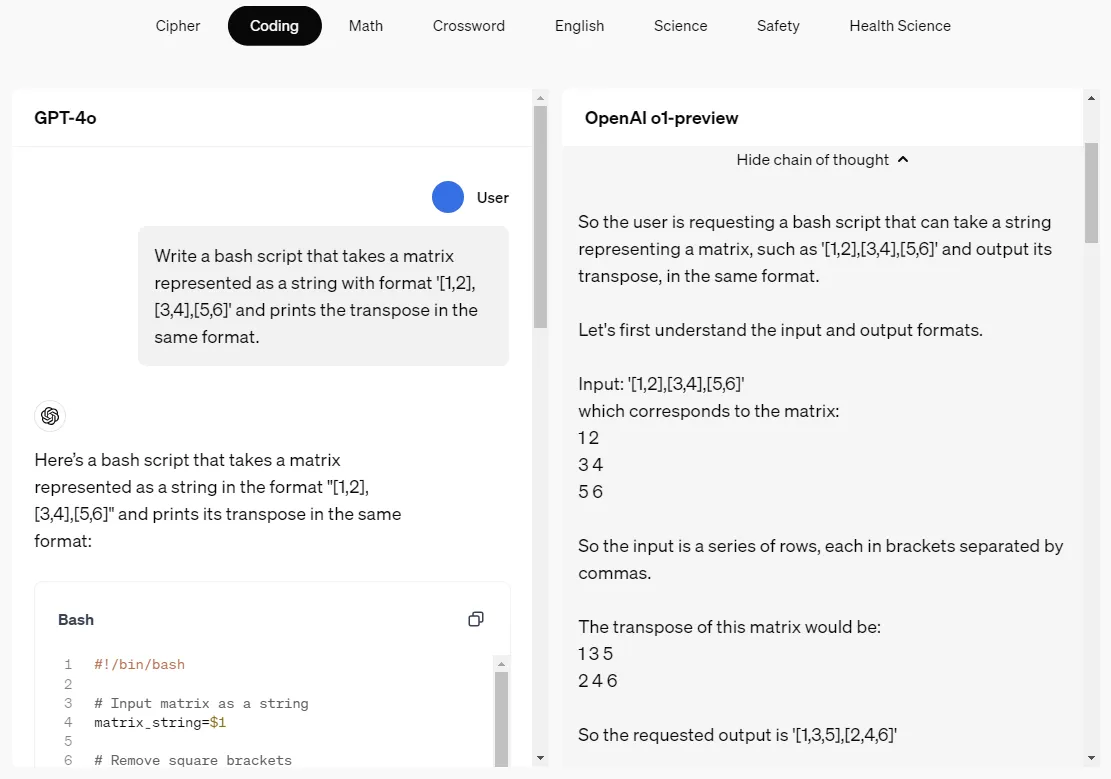
OpenAI o1-preview在编程场景下的思维链演示
OpenAI之前模型的思维模式是系统1(依赖直觉经验快速反馈),而o1模型的思维链则启动了系统2(耗费时间进行逻辑推理)。OpenAI采用大规模强化学习算法,训练o1模型如何在数据高度有效的训练过程中利用其思维链进行有效思考。o1模型像人类一样,用更多时间思考问题然后再做出反应。通过训练,模型学会完善自己的思维过程,尝试不同的策略,并认识到自己的错误。测试结果显示,o1模型在美国数学邀请赛(AIME)中的通过正确率,随着强化学习的增加(训练时间)和思考时间的增加(测试时间)而持续增加。OpenAI发现扩展此方法的限制与 LLM 预训练的限制大不相同,将继续研究这些限制。这一发现为Scaling Law赋予了新的内涵,即模型性能可以从强化学习的训练资源量投入和思维链思考时间两个维度进行提升。
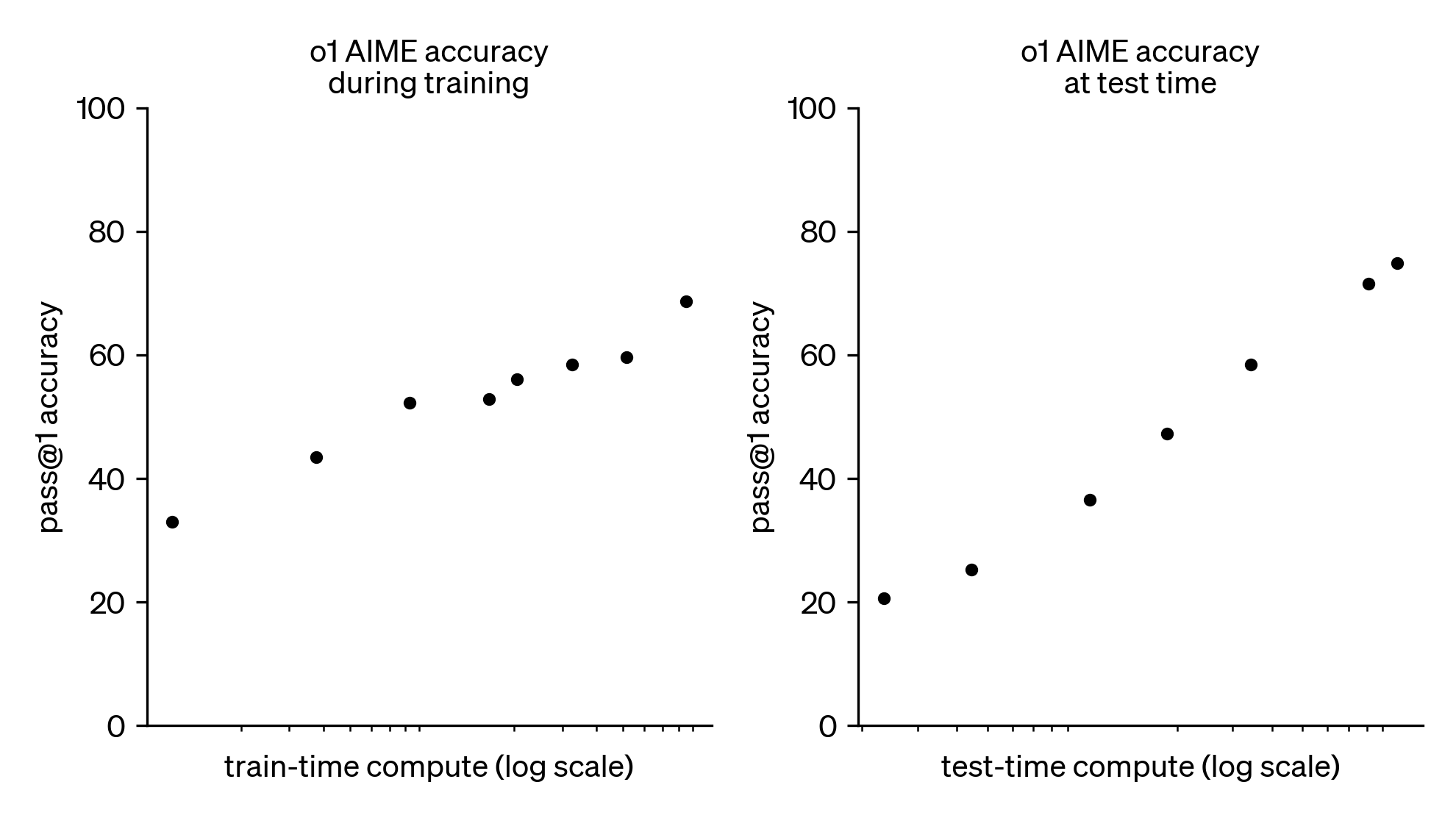
o1 性能随着训练时间和测试时间计算而平稳提升(规模采用对数)
以编程场景为例,Open AI o1为编程带来了更多的可能性。模型在 2024 年国际信息学奥林匹克 (IOI) 比赛中以213分获得49%分位的排名。对于每个问题,系统都会抽取许多候选提交,并根据测试时间选择策略提交其中的50个,如果随机提交,平均得分只有156 分。OpenAI发现,在放宽提交限制后,模型性能显著提高,当允许每个问题提交10,000次时,即使没有任何测试时间选择策略,该模型也能获得 362.14 分,高于金牌线。OpenAI还模拟了 Codeforces 主办的竞争性编程竞赛,以展示该模型的编码技术,GPT-4o的成绩仅位于人类参赛者的11%分位,而o1模型的 Elo 评分排名显著提升至89%分位,并通过微调后表现优于 93% 的人类竞争对手。
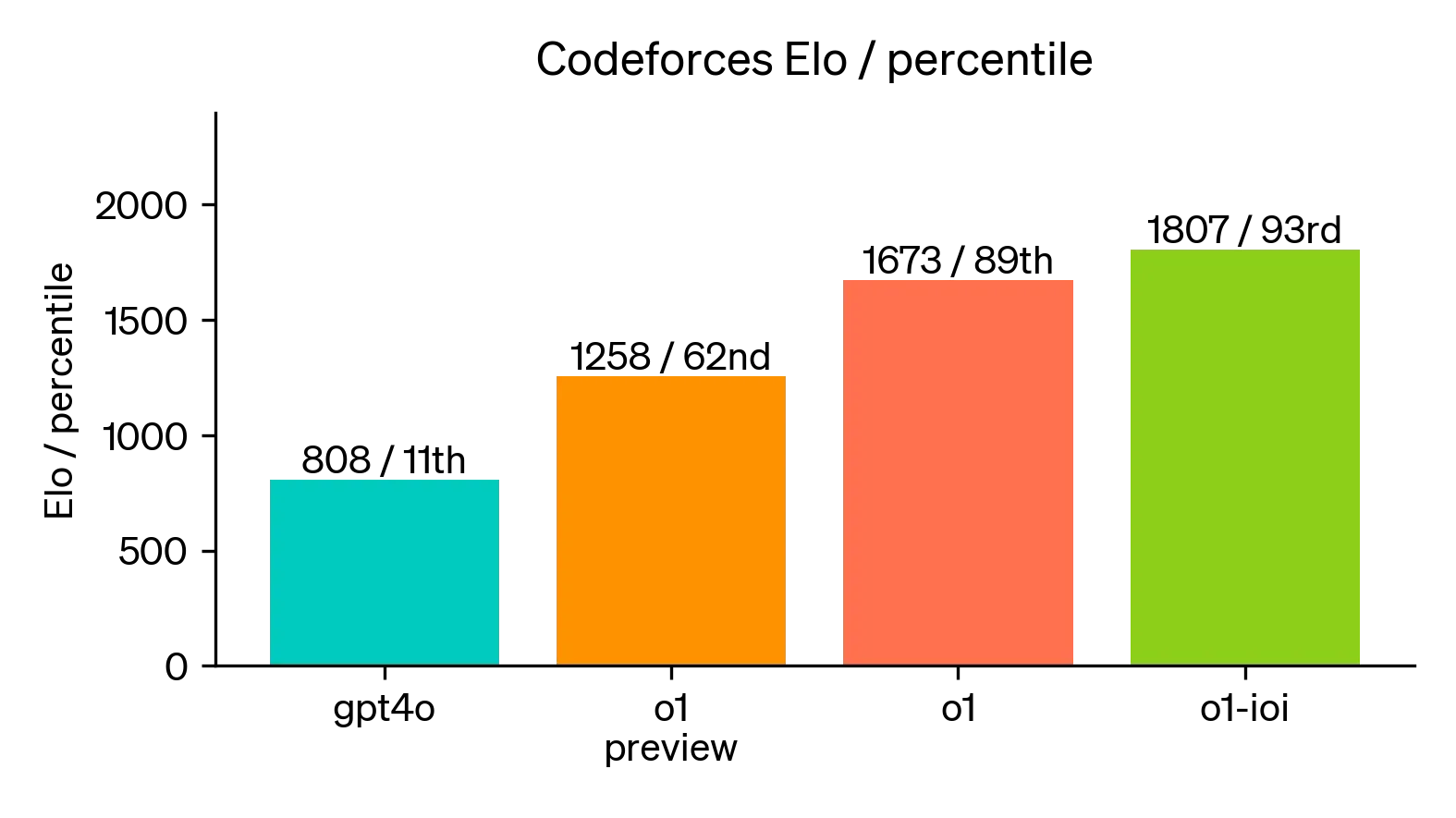
ioi通过进一步微调,提升了o1在2024年国际信息学奥林匹克竞赛规则下的排名
目前,OpenAI o1模型可通过网页版,或者是 API 进行访问。 对于开发者而言,使用OpenAI o1的成本十分昂贵。相比之下,GPT-4o的定价为输入5.00美元/百万 token,输出15.00美元/百万 token,GPT-4o mini的定价为输入0.15美元/百万 token,输出0.60美元/百万 token,同时,o1模型的思维链过程所消耗的token同样被计入输出token,这意味着输出的费用将进一步大幅提升。
OpneAI o1-preview是针对需要广泛常识的复杂任务而设计的新推理模型,具有 128K 上下文和截止2023年10月的知识,其定价为:
- 输入:15.00美元/百万token
- 输出:60.00美元/百万token
Open AI o1-mini是快速、经济高效的推理模型,专门针对编程、数学和科学领域。该模型具有 128K 上下文和截止2023年10月的知识,其定价为:
- 输入:3.00美元/百万token
- 输出:12.00美元/百万token
大模型领域的开源代码数据集有哪些
OpneAI o1强大的推理能力,预示着新一轮的大模型军备竞赛即将开启。o1模型在科学、编程和数学方面展现出复杂任务的推理能力,可以预见代码能力将成为评价大模型质量的重要因素。模型能力的提升需要高度有效的数据集作为训练基础,数据集的数量和质量极大影响着大规模强化学习算法的训练。
我们在上一篇技术文章中介绍了一系列用于提升大模型数学能力的开源数学数据集。这次我们将目光转向另一个同样重要的领域:模型的代码能力。代码数据集不仅需要涵盖不同的编程语言和范式,还要包含各种难度和类型的编程任务,以全面评估和提升模型的逻辑推理能力。
下面,我们将介绍一些在大模型代码能力训练和评估中广泛使用的开源代码数据集。
数据集名称 | 发布方 | 发布时间 | 数量 | 生成方式 | 语言 | 主要用途 |
---|---|---|---|---|---|---|
HumanEval | OpenAI | 2021 | 164个问题 | 人工编写 | Python | 代码生成评估 |
CodeSearchNet | GitHub | 2019 | 2m | 开源代码库抓取 | Python、Java、Php、Ruby、JavaScript、Go | 代码搜索、理解 |
CodeXGLUE | Microsoft | 2020 | - | 多种方式 | 多语言 | 综合代码智能评估 |
CodeNet | IBM Research | 2021 | 14m个提交 | 编程竞赛提交 | 55种语言 | 代码相似性、翻译 |
MBPP | Google Research | 2022 | 974个问题 | 人工编写 | Python | 基础编程能力评估 |
APPS | UC Berkeley | 2021 | 10,000个问题 | 编程竞赛题目 | Python | 算法问题解决能力评估 |
NaturalCodeBench | THUDM | 2023 | 402个问题 | 人工挑选 | Python、Java | 综合代码能力评估 |
The Stack v2 | BigCode | 2023 | 4.5TB | 开源代码库抓取 | 658种语言 | 大规模模型训练 |
HumanEval-x | THUDM | 2022 | 820个问题 | 人工编写 | Python、C++、Java、JavaScript、Go | 代码生成、翻译 |
1. Human-eval
https://github.com/openai/human-eval
{
"task_id": "HumanEval/0",
"prompt": "from typing import List\n\n\ndef has_close_elements(numbers: List[float], threshold: float) -> bool:\n \"\"\" Check if in given list of numbers, are any two numbers closer to each other than\n given threshold.\n >>> has_close_elements([1.0, 2.0, 3.0], 0.5)\n False\n >>> has_close_elements([1.0, 2.8, 3.0, 4.0, 5.0, 2.0], 0.3)\n True\n \"\"\"\n",
"entry_point": "has_close_elements",
"canonical_solution": " for idx, elem in enumerate(numbers):\n for idx2, elem2 in enumerate(numbers):\n if idx != idx2:\n distance = abs(elem - elem2)\n if distance < threshold:\n return True\n\n return False\n",
"test": "\n\nMETADATA = {\n 'author': 'jt',\n 'dataset': 'test'\n}\n\n\ndef check(candidate):\n assert candidate([1.0, 2.0, 3.9, 4.0, 5.0, 2.2], 0.3) == True\n assert candidate([1.0, 2.0, 3.9, 4.0, 5.0, 2.2], 0.05) == False\n assert candidate([1.0, 2.0, 5.9, 4.0, 5.0], 0.95) == True\n assert candidate([1.0, 2.0, 5.9, 4.0, 5.0], 0.8) == False\n assert candidate([1.0, 2.0, 3.0, 4.0, 5.0, 2.0], 0.1) == True\n assert candidate([1.1, 2.2, 3.1, 4.1, 5.1], 1.0) == True\n assert candidate([1.1, 2.2, 3.1, 4.1, 5.1], 0.5) == False\n\n"
}
Human-eval
数据集是由OpenAI
开发的一个用于评估代码生成模型能力的基准测试集。这个数据集包含164个原始的Python
编程问题,每个问题都包括一个函数签名、文档字符串(描述函数功能)、一个可运行的测试用例以及一个规范的参考实现。
Human-eval
提供了高质量、多样化的编程任务,主要应用于对代码生成模型的功能完整性和正确性进行严格评估。Human-eval
采用了"通过率"(pass@k)指标评估模型,这个指标衡量模型在生成k个候选解决方案时至少有一个通过所有测试用例的概率。这种评估方法更接近实际编程场景,因为它考虑到了代码需要在功能上完全正确,而不仅仅是看起来合理。
2. CodeSearchNet
https://github.com/github/CodeSearchNet
{
"repo": "soimort/you-get",
"path": "src/you_get/extractors/youtube.py",
"func_name": "YouTube.get_vid_from_url",
"original_string": "def get_vid_from_url(url):\n \"\"\"Extracts video ID from URL.\n \"\"\"\n return match1(url, r\'youtu\\\\.be/([^?/]+)\') or \\\\\\n match1(url, r\'youtube\\\\.com/embed/([^/?]+)\') or \\\\\\n match1(url, r\'youtube\\\\.com/v/([^/?]+)\') or \\\\\\n match1(url, r\'youtube\\\\.com/watch/([^/?]+)\') or \\\\\\n parse_query_param(url, \'v\') or \\\\\\n parse_query_param(parse_query_param(url, \'u\'), \'v\')",
"language": "python",
"code": "def get_vid_from_url(url):\n \"\"\"Extracts video ID from URL.\n \"\"\"\n return match1(url, r\'youtu\\\\.be/([^?/]+)\') or \\\\\\n match1(url, r\'youtube\\\\.com/embed/([^/?]+)\') or \\\\\\n match1(url, r\'youtube\\\\.com/v/([^/?]+)\') or \\\\\\n match1(url, r\'youtube\\\\.com/watch/([^/?]+)\') or \\\\\\n parse_query_param(url, \'v\') or \\\\\\n parse_query_param(parse_query_param(url, \'u\'), \'v\')",
"code_tokens": ["def", "get_vid_from_url", "(", "url", ")", ":", "return", "match1", "(", "url", ",", "r\'youtu\\\\.be/([^?/]+)\'", ")", "or", "match1", "(", "url", ",", "r\'youtube\\\\.com/embed/([^/?]+)\'", ")", "or", "match1", "(", "url", ",", "r\'youtube\\\\.com/v/([^/?]+)\'", ")", "or", "match1", "(", "url", ",", "r\'youtube\\\\.com/watch/([^/?]+)\'", ")", "or", "parse_query_param", "(", "url", ",", "\'v\'", ")", "or", "parse_query_param", "(", "parse_query_param", "(", "url", ",", "\'u\'", ")", ",", "\'v\'", ")"],
"docstring": "Extracts video ID from URL.", "docstring_tokens": ["Extracts", "video", "ID", "from", "URL", "."], "sha": "b746ac01c9f39de94cac2d56f665285b0523b974",
"url": "https://github.com/soimort/you-get/blob/b746ac01c9f39de94cac2d56f665285b0523b974/src/you_get/extractors/youtube.py#L135-L143",
"partition": "test"
}
CodeSearchNet
数据集是一个用于评估代码搜索和理解能力的大规模基准数据集。这个数据集包含了来自GitHub
的数百万行代码和相关的自然语言描述,涵盖了多种编程语言,如Python
、JavaScript
、Go
、Java
、PHP
和Ruby
。
CodeSearchNet
的量级特点在于提供了大量的代码-文档对,每对都包含了代码片段及其对应的文档字符串或注释。
CodeSearchNet
支持对当前代码搜索和理解模型的能力进行诊断,并推动相关研究的进展。通过CodeSearchNet
,研究者们能够测试和提升模型在代码检索、代码-自然语言匹配以及代码理解等任务中的性能。尤其是在面对需要深入理解代码语义和自然语言描述之间关系的场景时,CodeSearchNet
能够作为一个有效的基准来评估和改进模型的表现。
3. CodeXGLUE
https://github.com/microsoft/CodeXGLUE
{
"code": "boolean function ( ) { return isParsed ; }",
"id": 0,
"nl": "check if details are parsed . concode_field_sep Container parent concode_elem_sep boolean isParsed concode_elem_sep long offset concode_elem_sep long contentStartPosition concode_elem_sep ByteBuffer deadBytes concode_elem_sep boolean isRead concode_elem_sep long memMapSize concode_elem_sep Logger LOG concode_elem_sep byte[] userType concode_elem_sep String type concode_elem_sep ByteBuffer content concode_elem_sep FileChannel fileChannel concode_field_sep Container getParent concode_elem_sep byte[] getUserType concode_elem_sep void readContent concode_elem_sep long getOffset concode_elem_sep long getContentSize concode_elem_sep void getContent concode_elem_sep void setDeadBytes concode_elem_sep void parse concode_elem_sep void getHeader concode_elem_sep long getSize concode_elem_sep void parseDetails concode_elem_sep String getType concode_elem_sep void _parseDetails concode_elem_sep String getPath concode_elem_sep boolean verify concode_elem_sep void setParent concode_elem_sep void getBox concode_elem_sep boolean isSmallBox"
}
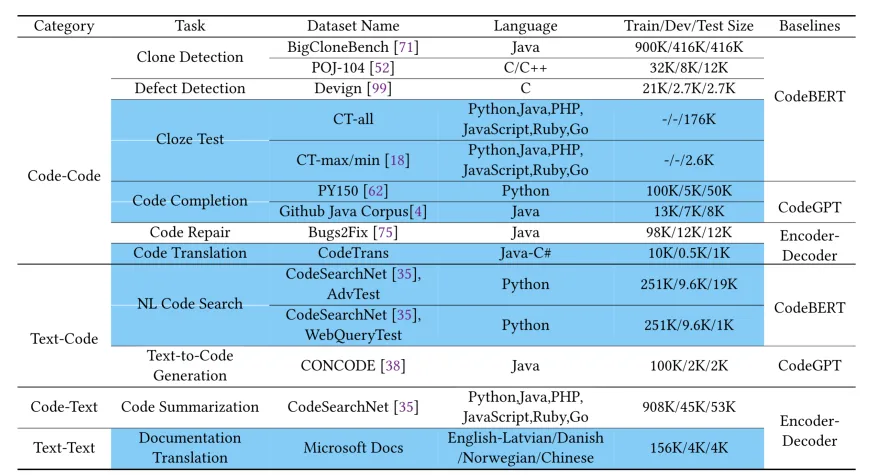
CodeXGLUE的10个子任务以及14个数据集
CodeXGLUE
数据集是由微软研究院开发的一个综合性代码智能基准测试集。这个数据集包含10个不同的代码智能任务,涵盖了代码-代码、文本-代码、代码-文本和代码-执行四大类别。每个任务都包括特定的数据集、评估指标和基准模型实现。CodeXGLUE
的特点在于它提供了多语言、多任务的评估框架,涵盖了从代码克隆检测到代码生成等多种常见的代码智能应用场景。
CodeXGLUE
的独特之处在于它提供了一个统一的评估框架,使得不同模型之间的性能可以直接比较。同时,它的多任务特性允许研究者评估模型的通用性和迁移学习能力。
4. Project_CodeNet
https://github.com/IBM/Project_CodeNet
while True:
try:
a, b = map(int, input().split(" "))
print(len(str(a + b)))
except:
break
Project CodeNet
是IBM Research
开发的大规模多用途数据集,用于推动代码智能领域的AI研究和应用。它包含超过1400万个代码样本,涵盖55种编程语言,总计约5亿行代码。
该数据集提供了大量问题-解决方案对,每个问题有多个解决方案,并包含丰富的元数据。 主要应用包括:
- 代码相似性分析
- 代码翻译
- 代码补全和生成
- 程序修复
- 计算复杂度估计
Project CodeNet
作为综合性基准,能评估和改进模型在各种代码智能任务中的表现,特别是在理解代码结构、语义和跨语言等价性方面。
5. the-stack-v2
https://huggingface.co/datasets/bigcode/the-stack-v2
{
"blob_id": "d44bbb217114c0831167824d694d57c29ab86665",
"directory_id": "e3f3f911019ac126d01c056eafc7c3183107a5af",
"path": "/Traffic Sign Detection/all_signs_combined/src/predict.py",
"content_id": "19ed9a428015b625610be9930dfee35938fb451b",
"repo_name": "uncctrafficsigndetection/Traffic-Sign-Detection",
"snpashot_id": "595258766f865c4b3c628b002d7b93a774168a9b",
"revision_id": "3ff4be52357f4b6340fef94124f8c835ab66fd8a",
"branch_name": "refs/heads/master",
"language": "Python",
"src_encoding": "UTF-8",
"is_generated": "false",
"length_bytes": 959
}
The Stack v2
是BigCode
项目开发的大规模多语言代码数据集。它包含来自GitHub
的开源代码,涵盖358多种编程语言,总计约4.5TB数据和2150亿个标记,是目前最大的公开代码数据集之一。
该数据集主要用于支持大规模语言模型在代码领域的训练和研究,包括:
- 训练专门的代码理解和生成模型
- 研究多语言代码表示和迁移学习
- 开发代码补全、搜索和翻译工具
- 探索代码结构和编程模式
- 研究代码注释和文档生成
The Stack v2
的独特之处在于其数据的多样性和规模,不仅包含常见编程语言,还包括许多不常见语言,为跨语言代码理解研究提供了宝贵资源。它能有效评估和改进模型在处理多种编程语言、理解复杂代码结构和语义方面的能力。
6. mbpp
https://huggingface.co/datasets/google-research-datasets/mbpp
{
"task_id":601,
"text":"Write a function to find the longest chain which can be formed from the given set of pairs.",
"code":"class Pair(object): def __init__(self, a, b): self.a = a self.b = b def max_chain_length(arr, n): max = 0 mcl = [1 for i in range(n)] for i in range(1, n): for j in range(0, i): if (arr[i].a > arr[j].b and mcl[i] < mcl[j] + 1): mcl[i] = mcl[j] + 1 for i in range(n): if (max < mcl[i]): max = mcl[i] return max",
"test_list":"[ 'assert max_chain_length([Pair(5, 24), Pair(15, 25),Pair(27, 40), Pair(50, 60)], 4) == 3", "assert max_chain_length([Pair(1, 2), Pair(3, 4),Pair(5, 6), Pair(7, 8)], 4) == 4", "assert max_chain_length([Pair(19, 10), Pair(11, 12),Pair(13, 14), Pair(15, 16), Pair(31, 54)], 5) == 5' ]",
"test_setup_code":'',
"challenge_test_list":[]
}
MBPP
是Google Research
开发的Python
编程问题集,包含974个原创问题,每个问题配有描述、解决方案代码和测试用例。它专注于基础到中级的Python
编程任务,涵盖多种编程概念和算法。
该数据集主要用于评估代码生成模型、改进模型性能、提供基准测试和支持编程教育。它能有效评估模型的自然语言理解、代码生成、语法正确性、功能完整性和算法实现能力。MBPP
的独特之处在于其专注于日常编程挑战,涵盖了字符串操作、列表处理、数学计算等实际编程中常见的任务类型。
研究人员可以利用MBPP
训练专门的Python
代码生成模型,评估大型语言模型在Python
任务上的表现,研究问题理解与代码生成的关系,以及开发智能编程辅助工具。
7. NaturalCodeBench
https://github.com/THUDM/NaturalCodeBench
{
"_id": 131,
"prompt": "你的任务是生成java代码来解决以下问题,生成的代码必须位于代码块```java和```之间,最多只允许有一个代码块:\n课堂练习:设计一个接受数组的静态方法,数组的每个成员都是个位整数(8到9之间),该方法能以电话号码的形式返回包含这些数字的字符串。例如:\n createPhoneNumber ( new int []{1,2,3,4,5,6,7,8,9,0})//=> returns \"(123) 456-7890\"\n注:别忘了右括号后面的空格!\n\n生成代码时你需要遵循以下测试用例中测试函数体中的函数名或类名,但生成的代码中不允许包含测例:\nclass MainTest {\n\n @Test\n void testCreatePhoneNumber1() {\n assertEquals(\"(123) 456-7890\", PhoneNumberCreator.createPhoneNumber(new int[]{1,2,3,4,5,6,7,8,9,0}));\n }\n}",
"problem": "课堂练习:设计一个接受数组的静态方法,数组的每个成员都是个位整数(8到9之间),该方法能以电话号码的形式返回包含这些数字的字符串。例如:\n createPhoneNumber ( new int []{1,2,3,4,5,6,7,8,9,0})//=> returns \"(123) 456-7890\"\n注:别忘了右括号后面的空格!",
"testcases": "import org.junit.jupiter.api.Test;\nimport static org.junit.jupiter.api.Assertions.*;\n\n\nclass PhoneNumberCreatorTest {\n @Test\n void testCreatePhoneNumber2() {\n assertEquals(\"(987) 654-3210\", PhoneNumberCreator.createPhoneNumber(new int[]{9,8,7,6,5,4,3,2,1,0}));\n }\n\n @Test\n void testCreatePhoneNumber3() {\n assertEquals(\"(111) 111-1111\", PhoneNumberCreator.createPhoneNumber(new int[]{1,1,1,1,1,1,1,1,1,1}));\n }\n\n @Test\n void testCreatePhoneNumber4() {\n assertEquals(\"(999) 999-9999\", PhoneNumberCreator.createPhoneNumber(new int[]{9,9,9,9,9,9,9,9,9,9}));\n }\n\n @Test\n void testCreatePhoneNumber5() {\n assertEquals(\"(123) 456-7890\", PhoneNumberCreator.createPhoneNumber(new int[]{1,2,3,4,5,6,7,8,9,0}));\n }\n\n @Test\n void testCreatePhoneNumber6() {\n assertEquals(\"(000) 000-0000\", PhoneNumberCreator.createPhoneNumber(new int[]{0,0,0,0,0,0,0,0,0,0}));\n }\n\n //boundary cases\n @Test\n void testCreatePhoneNumber7() {\n assertEquals(\"(123) 456-7890\", PhoneNumberCreator.createPhoneNumber(new int[]{1,2,3,4,5,6,7,8,9,0}));\n }\n\n @Test\n void testCreatePhoneNumber8() {\n assertEquals(\"(000) 000-0000\", PhoneNumberCreator.createPhoneNumber(new int[]{0,0,0,0,0,0,0,0,0,0}));\n }\n\n @Test\n void testCreatePhoneNumber9() {\n assertEquals(\"(999) 999-9999\", PhoneNumberCreator.createPhoneNumber(new int[]{9,9,9,9,9,9,9,9,9,9}));\n }\n\n @Test\n void testCreatePhoneNumber10() {\n assertEquals(\"(123) 456-7890\", PhoneNumberCreator.createPhoneNumber(new int[]{1,2,3,4,5,6,7,8,9,0}));\n }\n}",
"setup_code": "",
"reference_solution": "```java\n\npublic class PhoneNumberCreator {\n public static String createPhoneNumber(int[] numbers) {\n StringBuilder phoneNumber = new StringBuilder(\"(\");\n for (int i = 0; i < numbers.length; i++) {\n if (i == 3) {\n phoneNumber.append(\") \");\n } else if (i == 6) {\n phoneNumber.append(\"-\");\n }\n phoneNumber.append(numbers[i]);\n }\n return phoneNumber.toString();\n }\n}\n\n```",
"classification": "算法和数据结构"
}
{
"_id": 131,
"response": "```java\n\npublic class PhoneNumberCreator {\n public static String createPhoneNumber(int[] numbers) {\n StringBuilder phoneNumber = new StringBuilder(\"(\");\n for (int i = 0; i < numbers.length; i++) {\n if (i == 3) {\n phoneNumber.append(\") \");\n } else if (i == 6) {\n phoneNumber.append(\"-\");\n }\n phoneNumber.append(numbers[i]);\n }\n return phoneNumber.toString();\n }\n}\n\n```"
}
{
"_id": 131,
"prompt": "你的任务是生成python代码来解决以下问题,生成的代码必须位于代码块```python和```之间,最多只允许有一个代码块:\n创建一个名为`word_count`的函数,该函数接受一个文件路径作为参数,读取文件内容,并统计文件中每个单词出现的次数。函数应返回一个字典,其中键是单词,值是该单词在文件中出现的次数。要求忽略单词的大小写,并移除标点符号。最后,按照单词出现的次数降序排列字典项。如果有多个单词出现次数相同,按照字母顺序升序排列。函数不需要处理文件不存在的情况。\n\n你需要遵循以下测试用例中测试函数体中的函数名或类名, 生成的代码中不允许包含任何测例:\nclass Testword_count:\n def test_word_count_basic_file(self, capfd, tmp_path):\n file_path = tmp_path / 'test_basic.txt'\n with open(file_path, 'w', encoding='utf-8') as file:\n file.write(\"This is a basic test file with some common words.\")\n word_count(file_path)\n captured = capfd.readouterr()\n assert \"'this': 1\\n'is': 1\\n'a': 1\\n'basic': 1\\n'test': 1\\n'file': 1\\n'with': 1\\n'some': 1\\n'common': 1\\n'words': 1\\n\" in captured.out\n\n\n",
"problem": "创建一个名为`word_count`的函数,该函数接受一个文件路径作为参数,读取文件内容,并统计文件中每个单词出现的次数。函数应返回一个字典,其中键是单词,值是该单词在文件中出现的次数。要求忽略单词的大小写,并移除标点符号。最后,按照单词出现的次数降序排列字典项。如果有多个单词出现次数相同,按照字母顺序升序排列。函数不需要处理文件不存在的情况。",
"testcases": "import string\nfrom collections import Counter\n\n\nclass Testword_count:\n def test_word_count_case_sensitive_file(self, capfd, tmp_path):\n file_path = tmp_path / 'test_case_sensitive.txt'\n with open(file_path, 'w', encoding='utf-8') as file:\n file.write(\"Case case CASE\")\n word_count(file_path)\n captured = capfd.readouterr()\n assert \"'case': 3\" in captured.out\n\n\n def test_word_count_punctuation_file(self, capfd, tmp_path):\n file_path = tmp_path / 'test_punctuation.txt'\n with open(file_path, 'w', encoding='utf-8') as file:\n file.write(\"This sentence has some punctuation, like commas and periods.\")\n word_count(file_path)\n captured = capfd.readouterr()\n assert \"'this': 1\\n'sentence': 1\\n'has': 1\\n'some': 1\\n'punctuation': 1\\n'like': 1\\n'commas': 1\\n'and': 1\\n'periods': 1\\n\" in captured.out\n\n\n def test_word_count_same_word_file(self, capfd, tmp_path):\n file_path = tmp_path / 'test_same_word_multiple_times.txt'\n with open(file_path, 'w', encoding='utf-8') as file:\n file.write(\"word word word word word\")\n word_count(file_path)\n captured = capfd.readouterr()\n assert \"'word': 5\" in captured.out\n\n def test_word_count_different_words_file(self, capfd, tmp_path):\n file_path = tmp_path / 'test_different_words_same_count.txt'\n with open(file_path, 'w', encoding='utf-8') as file:\n file.write(\"apple banana orange\")\n word_count(file_path)\n captured = capfd.readouterr()\n assert \"'apple': 1\\n'banana': 1\\n'orange': 1\\n\" in captured.out\n\n def test_word_count_nonexistent_file(self, capfd, tmp_path):\n file_path = tmp_path / 'nonexistent_file.txt'\n word_count(file_path)\n captured = capfd.readouterr()\n assert \"Error: File\" in captured.out\n\n def test_word_count_empty_file(self, capfd, tmp_path):\n file_path = tmp_path / 'test_empty_file.txt'\n open(file_path, 'w', encoding='utf-8').close() # 创建一个空文件\n word_count(file_path)\n captured = capfd.readouterr()\n assert captured.out.strip() == \"\" # 期望输出为空字符串\n\n",
"setup_code": "",
"reference_solution": "```python\nimport string\nfrom collections import Counter\n\n\ndef word_count(file_path):\n try:\n # 读取文件内容\n with open(file_path, 'r', encoding='utf-8') as file:\n content = file.read()\n\n # 移除标点符号并转换为小写\n translator = str.maketrans(\"\", \"\", string.punctuation)\n content = content.translate(translator).lower()\n\n # 使用 Counter 统计单词出现次数\n words = content.split()\n word_counter = Counter(words)\n\n # 按照出现次数降序排列\n sorted_word_count = sorted(word_counter.items(), key=lambda x: x[1], reverse=True)\n\n for word, count in sorted_word_count:\n print(f\"'{word}': {count}\")\n\n except FileNotFoundError:\n print(f\"Error: File '{file_path}' not found.\")\n```",
"classification": "算法和数据结构"
}
{
"_id": 131,
"response": "```python\nimport string\nfrom collections import Counter\n\n\ndef word_count(file_path):\n try:\n # 读取文件内容\n with open(file_path, 'r', encoding='utf-8') as file:\n content = file.read()\n\n # 移除标点符号并转换为小写\n translator = str.maketrans(\"\", \"\", string.punctuation)\n content = content.translate(translator).lower()\n\n # 使用 Counter 统计单词出现次数\n words = content.split()\n word_counter = Counter(words)\n\n # 按照出现次数降序排列\n sorted_word_count = sorted(word_counter.items(), key=lambda x: x[1], reverse=True)\n\n for word, count in sorted_word_count:\n print(f\"'{word}': {count}\")\n\n except FileNotFoundError:\n print(f\"Error: File '{file_path}' not found.\")\n```"
}
NaturalCodeBench
是由清华大学THUDM
开发的综合性代码智能基准测试集。它整合了多个现有的代码评估数据集,并引入了新的评估维度,旨在提供更全面、更自然的代码能力评估框架。 这个数据集主要用于全面评估代码模型能力、跨任务比较、指导模型改进,以及模拟真实编程场景。其独特特点包括多维度评估、代码自然度评估、跨数据集整合、难度分层、多语言支持、实际编程场景模拟,以及持续更新的设计。
NaturalCodeBench
使研究人员能够全面评估和比较代码智能模型,识别模型优劣势,探索提高代码生成自然度和实用性的方法,以及研究模型的跨语言和跨任务泛化能力。
8. humaneval-x
https://huggingface.co/datasets/THUDM/humaneval-x
{
"task_id": "Python/0",
"prompt": "from typing import List\n\n\ndef has_close_elements(numbers: List[float], threshold: float) -> bool:\n \"\"\" Check if in given list of numbers, are any two numbers closer to each other than\n given threshold.\n >>> has_close_elements([1.0, 2.0, 3.0], 0.5)\n False\n >>> has_close_elements([1.0, 2.8, 3.0, 4.0, 5.0, 2.0], 0.3)\n True\n \"\"\"\n",
"declaration": "from typing import List\n\n\ndef has_close_elements(numbers: List[float], threshold: float) -> bool:\n",
"canonical_solution": "for idx, elem in enumerate(numbers):\n for idx2, elem2 in enumerate(numbers):\n if idx != idx2:\n distance = abs(elem - elem2)\n if distance < threshold:\n return True\n\n return False\n",
"test": "\n\nMETADATA = {\n 'author': 'jt',\n 'dataset': 'test'\n}\n\n\ndef check(has_close_elements):\n assert has_close_elements([1.0, 2.0, 3.9, 4.0, 5.0, 2.2], 0.3) == True\n assert has_close_elements([1.0, 2.0, 3.9, 4.0, 5.0, 2.2], 0.05) == False\n assert has_close_elements([1.0, 2.0, 5.9, 4.0, 5.0], 0.95) == True\n assert has_close_elements([1.0, 2.0, 5.9, 4.0, 5.0], 0.8) == False\n assert has_close_elements([1.0, 2.0, 3.0, 4.0, 5.0, 2.0], 0.1) == True\n assert has_close_elements([1.1, 2.2, 3.1, 4.1, 5.1], 1.0) == True\n assert has_close_elements([1.1, 2.2, 3.1, 4.1, 5.1], 0.5) == False\n\ncheck(has_close_elements)",
"text": "Check if in given list of numbers, are any two numbers closer to each other than\n given threshold.\n >>> has_close_elements([1.0, 2.0, 3.0], 0.5)\n False\n >>> has_close_elements([1.0, 2.8, 3.0, 4.0, 5.0, 2.0], 0.3)\n True",
"example_test": "def check(has_close_elements):\n assert has_close_elements([1.0, 2.0, 3.0], 0.5) == False\n assert has_close_elements([1.0, 2.8, 3.0, 4.0, 5.0, 2.0], 0.3) == True\ncheck(has_close_elements)\n"
}
HumanEval-X
是由清华大学THUDM
开发的多语言代码生成评估数据集,是对原始HumanEval
的扩展。它包含164个编程问题,每个问题有6种语言(Python
、C++
、Java
、JavaScript
、Go
和Rust
)的实现,共984个任务。
HumanEval-X
主要用于评估模型的多语言代码生成能力、跨语言迁移学习能力,以及语言无关的编程能力。它的独特之处在于多语言并行实现、保持原始难度、确保跨语言等效性,并提供自动评估脚本。
9. Automated Programming Progress Standard(APPS)
https://github.com/hendrycks/apps
{
'problem_id': 0,
'question': 'Polycarp has $n$ different binary words. A word called binary if it contains only characters \'0\' and \'1\'. For example...',
'solutions': ["for _ in range(int(input())):\n n = int(input())\n mass = []\n zo = 0\n oz = 0\n zz = 0\n oo = 0\n...",...],
'input_output': {'inputs': ['4\n4\n0001\n1000\n0011\n0111\n3\n010\n101\n0\n2\n00000\n00001\n4\n01\n001\n0001\n00001\n'],
'outputs': ['1\n3 \n-1\n0\n\n2\n1 2 \n']},
'difficulty': 'interview',
'url': 'https://codeforces.com/problemset/problem/1259/D',
'starter_code': ''}
}
Automated Programming Progress Standard (APPS)
是由UC Berkeley
研究人员开发的一个用于评估代码生成模型能力的大规模基准测试集。这个数据集包含了 10,000 个原创编程问题,涵盖了从基础到高级的各种难度级别,其中甚至包括一些编程竞赛级别的挑战性问题。
APPS
的每个问题都包括详细的问题描述、函数签名、测试用例和参考解决方案,这种结构化的数据设计使其成为评估代码生成模型的理想工具。虽然APPS
主要针对Python
语言,但它也支持其他编程语言,增加了其应用的灵活性。数据集还配备了自动化评估脚本,可以客观地测试生成代码的正确性,这大大简化了研究过程。APPS
的主要应用在于评估代码生成模型的综合能力,特别是在处理复杂和多样化编程任务时的表现。研究人员可以通过APPS
来测试模型理解问题描述并生成正确实现的能力,以及在不同难度级别任务中的表现。
11. DeepSeek-Coder
https://github.com/deepseek-ai/DeepSeek-Coder
deepseek
不仅在上一期提到的数学领域有优秀的表现,在代码领域也同样令人眼前一亮,本期依旧介绍deepseek-coder
的非开源数据集的生成流程

这个数据集主要由代码数据和指令数据两部分组成。代码数据来源于公开的代码仓库,特别是GitHub
,经过了严格的数据清洗和过滤流程,包括去除重复代码、低质量代码和可能包含个人信息的代码。指令数据则包括代码相关的问答对和多轮对话数据,部分来自对现有开源数据集的整理和筛选,另一部分是通过大型语言模型生成的合成数据,这些数据经过人工审核以确保质量。在数据处理过程中,DeepSeek-AI
使用tree-sitter
进行代码解析和标记化,应用特定的编码方案以保留代码的结构信息,并进行去重和质量控制。